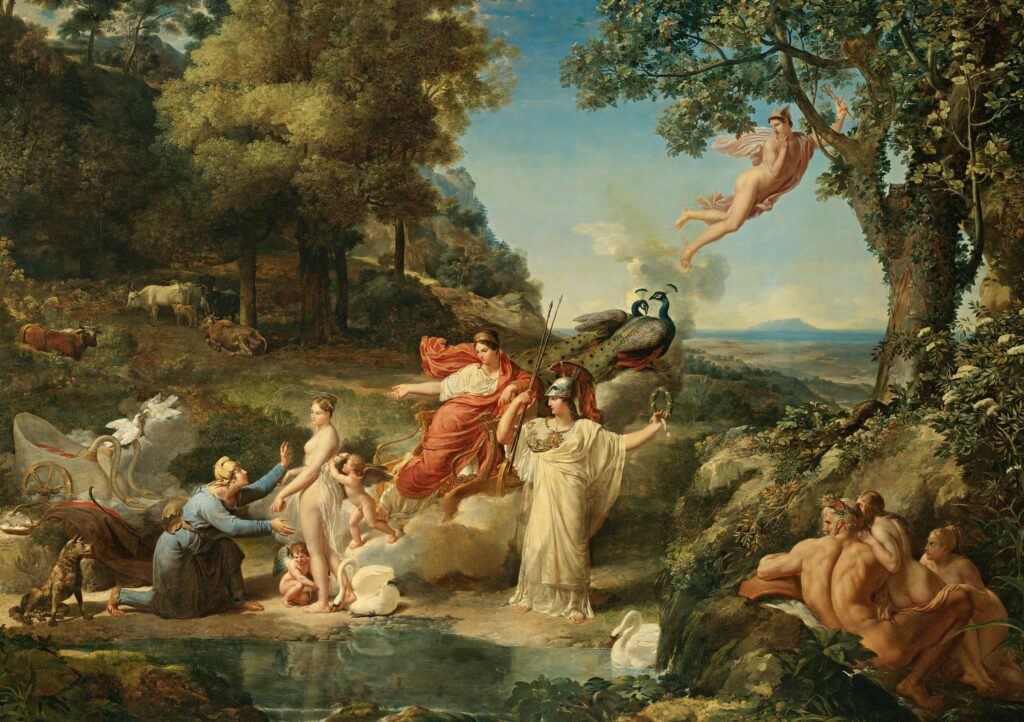
Epi Explained: Understanding Statistical Significance, P-Values, and Z-Scores
Statistical significance, p-values, and z-scores are fundamental concepts in statistics and research. They are crucial in fields such as medicine, psychology, and economics, helping researchers determine if their findings are due to chance or represent genuine relationships in the data. This Epi Explained aims to make these concepts more accessible by explaining them in detail and breaking down their importance in research.
Key Takeaways
What does a p-value of 0.05 mean?
A p-value of 0.05 means there’s a 5% chance that the results occurred due to random variation if the null hypothesis is true. If the p-value is lower than 0.05, the results are considered statistically significant.
What is the difference between a z-score and a p-value?
A z-score measures how far a data point is from the mean in terms of standard deviations, while a p-value quantifies the probability of obtaining the observed results if the null hypothesis is true.
Can a high p-value still be useful?
Yes. A high p-value suggests that the observed data could have occurred by chance, which means there might not be enough evidence to reject the null hypothesis. However, this can still provide valuable information, such as when a treatment shows no effect.
What Is Statistical Significance?
Statistical significance measures whether the results observed in a study are unlikely to have occurred by chance. It’s a key concept in hypothesis testing, which is a structured way to test ideas and assumptions in research. By determining statistical significance, researchers assess whether their findings support a particular hypothesis.
The Basics of Hypothesis Testing
Hypothesis testing involves two opposing statements:
- Null Hypothesis (H0): There is no effect or no difference between the groups being compared.
- Alternative Hypothesis (H1 or Ha): There is an effect or a difference between the groups.
The goal of hypothesis testing is to determine whether there is enough evidence to reject the null hypothesis in favor of the alternative hypothesis. Statistical significance helps to decide whether the observed data supports rejecting H0.
How to Calculate Statistical Significance
To calculate statistical significance, researchers use a measure called the p-value. The p-value represents the probability of observing results as extreme as (or more extreme than) those obtained, assuming the null hypothesis is true. A low p-value indicates that the observed results are unlikely under the null hypothesis, signaling statistical significance.
Understanding the P-Value
The p-value, or probability value, is a key element in hypothesis testing. It helps quantify how likely the data would occur if the null hypothesis were true.
Interpreting P-Values
- P-Value < 0.05: The results are considered statistically significant, meaning there’s less than a 5% probability that the observed data is due to random chance. Researchers may conclude that there is evidence in favor of the alternative hypothesis.
- P-Value ≥ 0.05: The results are not statistically significant. This suggests that any differences or effects observed could likely be due to chance, and researchers usually cannot reject the null hypothesis.
It’s essential to remember that a p-value does not measure the probability that the null hypothesis is true or false. Instead, it shows the probability of the data assuming the null hypothesis is true. Additionally, while 0.05 is commonly used as the threshold, many researchers opt for stricter cutoffs, such as 0.01, depending on the context of the study.
Concerns About Overusing P-Values
In some fields, especially biomedical research, the reliance on p-values has been criticized. A significant p-value doesn’t always mean the effect is practically meaningful or important. This has led to calls for more nuanced approaches in interpreting statistical findings.
What Is a Z-Score?
The z-score, also known as the standard score, measures how far a particular data point is from the mean of the dataset, expressed in terms of standard deviations. It’s a crucial concept for comparing different values and calculating probabilities in statistics.
Z-Score Formula
The formula for calculating the z-score is:
[math] z = (X − μ) / σ [/math]
Where:
- X is the value being measured.
- μ is the mean of the population.
- σ is the standard deviation of the population.
Interpreting the Z-Score
- Positive Z-Score: Indicates that the value is above the mean.
- Negative Z-Score: Indicates that the value is below the mean.
- The magnitude of the z-score (how large or small the number is) indicates how many standard deviations away the value is from the mean.
Real-World Application of Statistical Significance and Z-Scores
These concepts are widely used in research, particularly in clinical trials and other fields where decision-making relies on data. That said, even in day to day public health work, significance is frequency used to check the strength of association between health related outcomes and variables, determining if a cluster of healthcare encounters is to be seen as normal or a potential significant outbreak/issue, or to do program evaluation.
Example Problem: Drug Testing
Let’s say a study is testing whether a new drug lowers blood pressure. The null hypothesis states that the drug has no effect on blood pressure, while the alternative hypothesis suggests it does lower blood pressure.
After conducting the study with 100 patients, the researchers find that the drug reduces blood pressure by an average of 5 mmHg, with a p-value of 0.02. This indicates that there is only a 2% chance that this result could have occurred by chance if the drug had no effect, making the results statistically significant.
If the mean blood pressure change in the population is 0 mmHg, and the standard deviation is 10 mmHg, the z-score for the observed decrease can be calculated as:
[math] z = (5 − 0) / 10 = 0.5 [/math]
This means the observed blood pressure decrease is 0.5 standard deviations above the mean expected under the null hypothesis, providing additional support for rejecting the null hypothesis.
Conclusion
Statistical significance, p-values, and z-scores are essential tools for analyzing data and drawing valid conclusions in research. By understanding these concepts, you can assess whether observed effects are likely real or the result of random variation. Whether you’re involved in research or simply trying to understand scientific studies, grasping these ideas is key to making data-driven decisions.
Humanities Moment
The featured image for this Epi Explained is The Judgement Of Paris (1812) by Guillaume-Guillon Lethière (French, 1760 – 1832). Guillaume Guillon-Lethière, a French neoclassical painter born in Guadeloupe in 1760, was the son of a French colonial official and a “mulatto” mother. He studied under Gabriel François Doyen, competed in the Prix de Rome, and established a successful studio in Paris, rivaling Jacques-Louis David. Elected to the Académie and awarded the Légion d’honneur in 1818, he later became a professor at the École des Beaux-Arts, mentoring students who would often cultivate a reputation of their own.
As always, thank you for reading this installment of Epi Explained. If you’d like to learn more about Epidemiology, Public Health, or the skills needed to excel in it, please check out our other articles here.