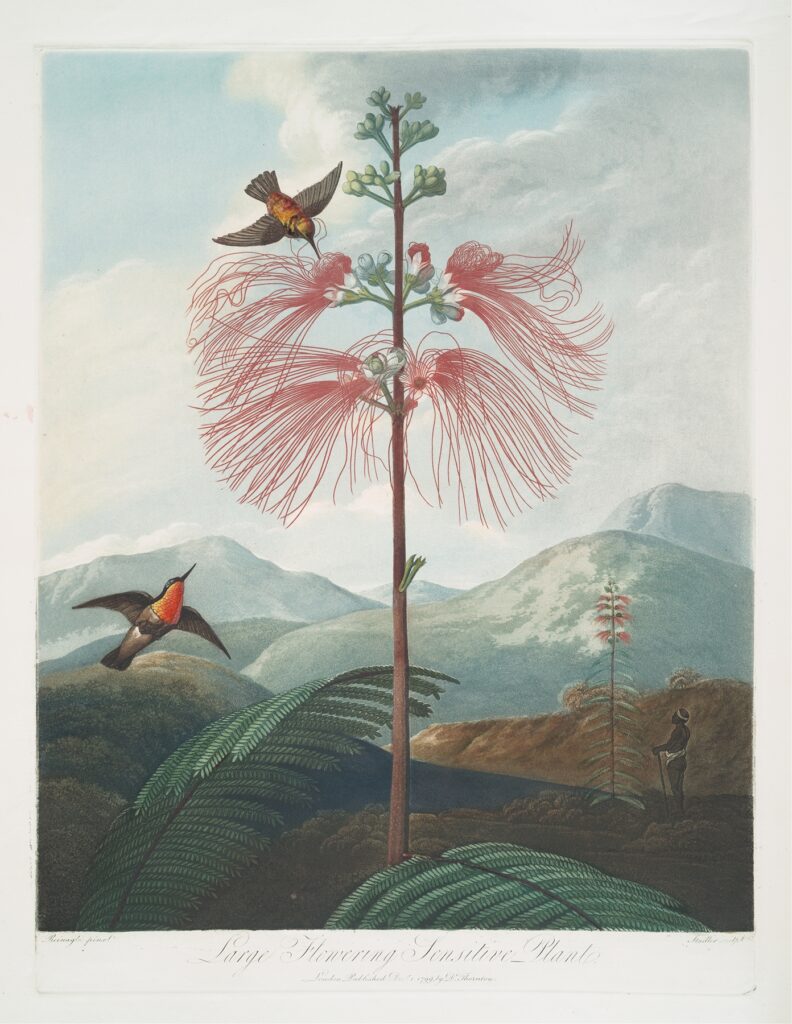
Epi Explained: What are Sensitivity and Specificity in Epidemiology?
Quick Takeaways
- Sensitivity measures a test’s ability to correctly identify individuals with the disease.
- Specificity measures how well a test correctly identifies individuals without the disease.
- Sensitivity and specificity are key in reducing false positives and negatives, crucial for public health screening.
- Predictive values (PPV and NPV) depend on the prevalence of the disease in the population.
- Advances in diagnostic technology aim to improve the balance between sensitivity and specificity.
Sensitivity and specificity are two of the most critical metrics in epidemiology, essential for analyzing the accuracy of diagnostic tests and the effectiveness of public health screening programs. Whether you’re a public health professional, a student, or someone with a general interest in public health, understanding these concepts is vital for interpreting data and making informed decisions. This article delves into the core definitions of sensitivity and specificity, explains their real-world applications, and explores their importance in epidemiological analysis.
Understanding the Basics of Sensitivity and Specificity
Sensitivity: The Test’s Ability to Detect Disease
Sensitivity refers to a test’s ability to correctly identify individuals who have a specific disease. In epidemiological studies, a highly sensitive test ensures that most cases of the disease are correctly identified, reducing the likelihood of missed diagnoses. Sensitivity is mathematically expressed as:
[math] \text{Sensitivity} = \frac{ \text{True Positives} }{ \text{True Positives} + \text{False Negatives} } [/math]
This equation calculates the proportion of individuals who test positive among those who truly have the disease. For example, a test with a sensitivity of 90% will correctly identify 90% of individuals with the disease, but it may miss 10%, leading to false negatives.
Example: Consider a tuberculosis (TB) screening program in high-prevalence regions. A sensitive test, such as the tuberculin skin test, is crucial to ensuring that cases of TB are detected early to prevent transmission. However, high sensitivity can sometimes lead to more false positives, especially in populations vaccinated with BCG.
Specificity: The Test’s Accuracy in Identifying Healthy Individuals
Specificity is the ability of a test to correctly identify individuals who do not have the disease. This metric is important in minimizing false positives. Specificity is calculated as:
[math] \text{Specificity} = \frac{ \text{True Negatives} }{ \text{True Negatives} + \text{False Positives} } [/math]
A test with high specificity ensures that most healthy individuals are not incorrectly diagnosed with the disease. In epidemiological terms, specificity is important for confirming diagnoses and ensuring that healthy individuals are not misclassified as sick.
Example: In diagnosing HIV, tests with high specificity, such as confirmatory Western blot tests, are essential. These tests ensure that people who are not infected are not incorrectly diagnosed as HIV-positive, thus preventing unnecessary stress and treatment.
The Mathematical Foundations of Sensitivity and Specificity
Calculating Sensitivity
Sensitivity, as discussed earlier, is calculated by dividing the number of true positives by the sum of true positives and false negatives. This measure helps determine how effectively a test can identify individuals with the disease:
[math] \text{Sensitivity} = \frac{ \text{True Positives} }{ \text{True Positives} + \text{False Negatives} } [/math]
For example, if a test detects 120 true positive cases of a disease but misses 30 cases (false negatives), the sensitivity of the test is:
[math] \text{Sensitivity} = \frac{ 120 }{ 120 + 30 } = 0.80 [/math]
This means the test has an 80% sensitivity, meaning it detects 80% of the true positive cases.
Calculating Specificity
Specificity is calculated by dividing the number of true negatives by the sum of true negatives and false positives. This calculation ensures we can evaluate how well the test identifies healthy individuals:
[math] \text{Specificity} = \frac{ \text{True Negatives} }{ \text{True Negatives} + \text{False Positives} } [/math]
If a test correctly identifies 90 healthy individuals (true negatives) but misidentifies 10 healthy individuals as positive (false positives), the specificity would be:
[math] \text{Specificity} = \frac{ 90 }{ 90 + 10 } = 0.90 [/math]
This test has 90% specificity, meaning it correctly identifies 90% of healthy individuals.
Sensitivity and Specificity in Public Health Screening
The Role in Disease Control
In public health screening programs, sensitivity and specificity are crucial for disease control and prevention efforts. A sensitive test ensures that as many cases as possible are detected early, especially in high-prevalence conditions. Specificity, on the other hand, ensures that false positives are minimized, reducing unnecessary treatment and anxiety in healthy individuals.
Example: Consider diabetes screening. Tests like fasting glucose or HbA1c are used to detect the disease. A highly sensitive test ensures that most individuals with diabetes are caught early, but if specificity is too low, many individuals without diabetes may be incorrectly identified, leading to over-treatment and higher healthcare costs.
Balancing Sensitivity and Specificity for Optimal Screening
Balancing sensitivity and specificity is a significant challenge in public health screening programs. High sensitivity may lead to increased false positives, while high specificity may miss some true cases. For example, in cancer screening, such as mammography, balancing sensitivity (to catch as many cases as possible) and specificity (to reduce unnecessary biopsies) is critical to effective screening and healthcare system efficiency.
Sensitivity and Specificity in Predictive Values
Positive Predictive Value (PPV)
Positive predictive value (PPV) is the probability that a person with a positive test result truly has the disease. PPV is influenced by both the sensitivity and specificity of the test as well as the prevalence of the disease in the population. It is calculated as:
[math] \text{PPV} = \frac{ \text{True Positives} }{ \text{True Positives} + \text{False Positives} } [/math]
Example: If a population has a low prevalence of disease, even a test with high specificity may produce a significant number of false positives, reducing the PPV.
Negative Predictive Value (NPV)
Negative predictive value (NPV) is the probability that a person with a negative test result truly does not have the disease. Similar to PPV, NPV is affected by the prevalence of the disease. It is calculated as:
[math] \text{NPV} = \frac{ \text{True Negatives} }{ \text{True Negatives} + \text{False Negatives} } [/math]
For instance, in a population where the disease is rare, a test with high NPV is highly reliable in indicating that a negative result is truly negative.
Real-World Applications of Sensitivity and Specificity
Case Study: Sensitivity and Specificity in HIV Testing
HIV testing is an excellent example of how sensitivity and specificity are used to make crucial health decisions. Initial HIV screening tests, like enzyme immunoassays (EIAs), are designed to be highly sensitive, ensuring that nearly all HIV-positive cases are detected. Confirmatory tests, such as the Western blot, are then used to ensure high specificity, ruling out false positives.
Challenges in Achieving High Sensitivity and Specificity
While it is desirable to have tests with both high sensitivity and specificity, in reality, trade-offs are often made depending on the context. In some cases, public health programs may prioritize sensitivity to ensure that as many cases as possible are identified. In other situations, specificity may be prioritized to reduce unnecessary treatments and resource use. Lastly, there are occasions where the condition or disease itself when combined with volume and community can make it hard to establish a baseline for sensitivity or specificity.
Sensitivity and Specificity in Decision-Making
Sensitivity and Specificity in Clinical Decision-Making
Healthcare providers rely on sensitivity and specificity metrics to inform clinical decision-making. For example, in deciding whether to proceed with invasive diagnostic procedures, a highly specific test can help confirm the necessity of such measures, reducing patient risk and healthcare costs.
Public Health Policies and Sensitivity/Specificity
At the population level, public health policies often rely on balancing sensitivity and specificity to create effective screening guidelines. This balance ensures that healthcare resources are used efficiently while maximizing the health benefits of screening programs.
Common Misconceptions about Sensitivity and Specificity
Misinterpretation of Test Results
One common misconception is that high sensitivity or specificity alone ensures a perfect test. In reality, these metrics must be considered alongside other factors like disease prevalence and predictive values. For example, a test with high sensitivity but low specificity may result in many false positives, leading to unnecessary follow-up testing and treatment.
Confusing Sensitivity/Specificity with Predictive Values
It is essential to understand that sensitivity and specificity are not the same as positive predictive value (PPV) and negative predictive value (NPV). Sensitivity and specificity are intrinsic properties of the test itself, whereas PPV and NPV depend on the disease prevalence in the population being tested.
Improving Sensitivity and Specificity in Future Testing
Innovations in Test Design
Advances in diagnostic technology, such as machine learning algorithms and molecular diagnostics, are helping to improve both the sensitivity and specificity of tests. These technologies allow for more precise detection and classification of diseases, which is particularly valuable in the context of rare or complex diseases.
The Future of Public Health Diagnostics
As public health continues to advance, new diagnostic tools will enable better screening programs. These innovations will likely focus on optimizing sensitivity and specificity to ensure accurate diagnosis while minimizing false results. Such advancements are crucial for improving healthcare efficiency and patient outcomes globally.
Conclusion
Sensitivity and specificity are fundamental concepts in epidemiology, essential for understanding and interpreting diagnostic tests and public health screening programs. While both metrics are critical for determining test accuracy, they must be used in conjunction with other factors, such as disease prevalence and predictive values, to make informed public health decisions. As diagnostic technology continues to evolve, we can expect improvements in both sensitivity and specificity, leading to better health outcomes and more efficient use of healthcare resources.
For public health professionals and students, understanding these metrics is crucial for interpreting data and making sound decisions that affect population health. As testing continues to play a central role in disease prevention and control, sensitivity and specificity will remain at the heart of effective epidemiological analysis and decision-making.
Humanities Moment
The featured article for this image is Large Flowering Sensitive Plant. (1799–1807) by Robert John Thornton (English, 1768-1837). Robert John Thornton was an English physician and botanical writer, best known for his work “A New Illustration of the Sexual System of Carolus von Linnæus” (1797-1807), which included the celebrated “Temple of Flora.” Inspired by the work of Linnaeus and John Martyn’s lectures, he transitioned from studying theology to medicine, later practicing and lecturing in medical botany in London.
Thank you for reading this installment of EpiExplained. If you’re interested in learning more about Epidemiology, check out our other Epi Explained articles, or look at our other tutorials, research summaries, or software!