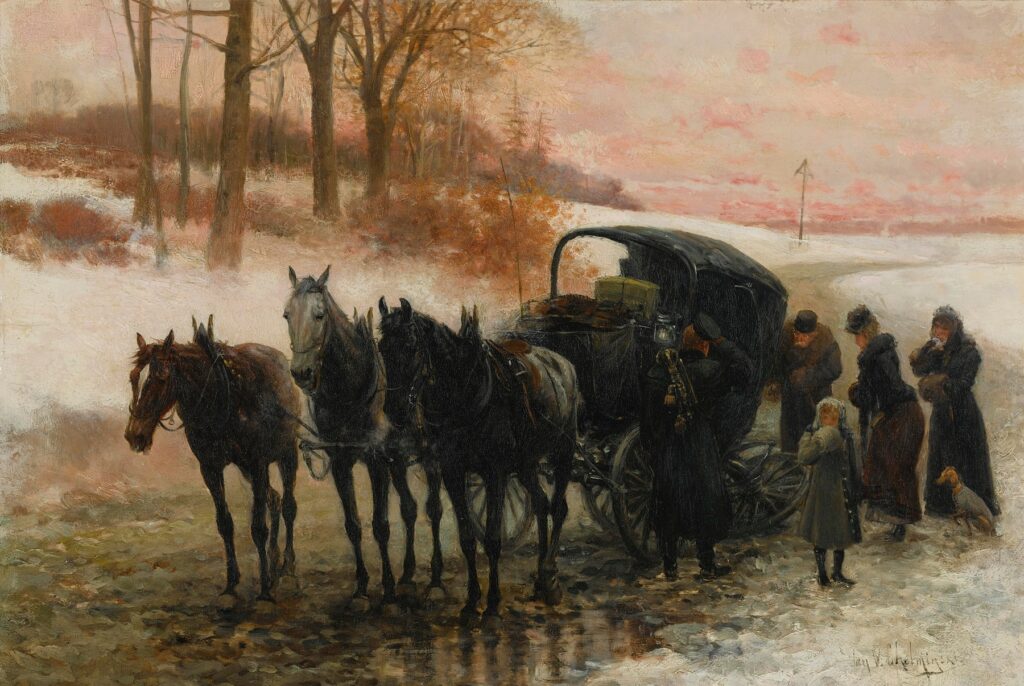
Epi Explained: Understanding Biases and Errors in Epidemiology
Quick Takeaways
- What is the difference between bias and random error in epidemiology? Bias is a systematic deviation from the truth, while random error occurs by chance. Random error can be reduced by increasing the sample size, but bias requires methodological changes to correct.
- How does selection bias affect a study? Selection bias arises when the participants in a study are not representative of the general population, potentially skewing the study’s findings.
- What is a confounder in epidemiology? A confounder is a variable that affects both the exposure and the outcome, distorting the observed relationship between them.
- What is the role of logistic regression in controlling confounding? Logistic regression is a statistical tool used to adjust for confounders by including them as variables in the regression model. This approach allows for the estimation of the association between the primary exposure and the outcome while controlling for the potential confounding effects of other variables. By incorporating both the exposure of interest and confounding variables as predictors in the model, logistic regression helps isolate the true effect of the exposure on the outcome.
Introduction to Biases and Errors in Epidemiology
In the field of epidemiology, ensuring the accuracy and reliability of research is vital for understanding health patterns and guiding public health decisions. Biases and errors—whether systematic or random—can distort study results, leading to inaccurate conclusions and possibly flawed public health policies. This article will explore key concepts related to biases and errors, including their mathematical underpinnings, and offer strategies for minimizing these issues in epidemiological research.
What is Bias in Epidemiology?
Bias in epidemiology refers to systematic errors that consistently skew the results of a study away from the true value. Unlike random errors, which occur by chance and can be reduced with larger sample sizes, biases are inherent to the study’s design or methodology.
Types of Bias
- Systematic Error: A consistent error in measurement or methodology leading to a biased estimate of effect.
- Random Error: Variability that occurs by chance and can be minimized through proper statistical techniques.
Mathematical Representation of Bias
Bias is often quantified using mathematical tools. For instance, the bias (B) of an estimator is the difference between the expected value of the estimator and the true value of the parameter being estimated:
[math] B(\hat{\theta}) = E[\hat{\theta}] – \theta [/math]
Where:
- [math] \hat{\theta} [/math] is the estimator,
- [math] \theta [/math] is the true value,
- [math] E[\hat{\theta}] [/math] represents the expected value of the estimator.
If the bias is zero, the estimator is considered unbiased.
Types of Bias in Epidemiology
Selection Bias
Selection bias arises when the participants selected for a study are not representative of the target population, which leads to skewed estimates. This often happens when certain groups are more likely to be included in the study than others.
Example: Case-Control Studies
In a case-control study, if certain cases are more likely to participate due to specific characteristics (e.g., accessibility to healthcare), the study may overestimate or underestimate the association between the exposure and the outcome.
Mathematical Illustration
The bias from selection can distort measures like the odds ratio (OR), calculated as:
[math] OR = \frac{(a/c)}{(b/d)} = \frac{a \times d}{b \times c} [/math]
Where:
- [math] a [/math] = number of exposed individuals with the outcome,
- [math] b [/math] = number of exposed individuals without the outcome,
- [math] c [/math] = number of unexposed individuals with the outcome,
- [math] d [/math] = number of unexposed individuals without the outcome.
Information Bias
Information bias occurs when there is misclassification of exposure or outcome status due to errors in measurement. This can result from poor recall in interviews or inconsistencies in diagnostic testing.
Recall Bias
For instance, in recall bias, individuals with a disease may remember exposures more clearly than those without the disease, which can distort study findings.
Misclassification Error
Misclassification can be either differential or non-differential. Differential misclassification occurs when errors depend on the outcome or exposure status, often pushing the results in a particular direction. Non-differential misclassification usually biases results toward the null hypothesis.
Confounding
Confounding occurs when a third variable, the confounder, distorts the relationship between the exposure and the outcome. The confounder is associated with both the exposure and the outcome but is not part of the causal chain. This can lead to incorrect conclusions about the effect of the exposure on the outcome.
Example of Confounding: Air Pollution and Asthma
Imagine studying the relationship between air pollution (the exposure) and asthma (the outcome). Socioeconomic status (SES) could be a confounder if people of lower SES are more likely to live in polluted areas and also more likely to develop asthma for reasons unrelated to air pollution.
Mathematical Explanation of Confounding
To quantify confounding, epidemiologists calculate both a crude odds ratio and an adjusted odds ratio. The crude OR does not account for confounding, while the adjusted OR controls for confounding factors like SES.
Crude Odds Ratio
[math] OR = \frac{(a/c)}{(b/d)} = \frac{a \times d}{b \times c} [/math]
Where:
- [math] a [/math] = number of exposed individuals with the outcome,
- [math] b [/math] = number of exposed individuals without the outcome,
- [math] c [/math] = number of unexposed individuals with the outcome,
- [math] d [/math] = number of unexposed individuals without the outcome.
Adjusted Odds Ratio Using Logistic Regression
To adjust for confounding, logistic regression models include the confounder in the equation:
[math] \log(OR) = \beta_0 + \beta_1 X + \beta_2 Z [/math]
Where:
- [math] \beta_0 [/math] is the intercept,
- [math] \beta_1 [/math] represents the effect of the exposure [math] X [/math],
- [math] \beta_2 [/math] represents the effect of the confounder [math] Z [/math].
Case Study of Bias and Error: Coffee Consumption and Heart Disease
Consider the relationship between coffee consumption and heart disease. Early studies suggested that coffee drinkers had higher rates of heart disease. However, when researchers adjusted for smoking—a known risk factor for heart disease—they found that the relationship between coffee consumption and heart disease was much weaker. Smoking acted as a confounder because it was associated with both coffee drinking and heart disease.
Effect of Confounding
The crude odds ratio (before adjusting for smoking) showed a strong association, but the adjusted odds ratio (after adjusting for smoking) demonstrated that the original association was largely due to confounding.
Crude Odds Ratio
[math] OR_{\text{crude}} = \frac{(a/c)}{(b/d)} = 3.5 [/math]
This suggests a 3.5 times higher risk of heart disease among coffee drinkers.
Adjusted Odds Ratio
After controlling for smoking:
[math] OR_{\text{adjusted}} = 1.2 [/math]
After accounting for smoking, the risk associated with coffee consumption was much smaller, indicating that smoking was a confounder.
Conclusion
Biases and errors can significantly impact the accuracy of epidemiological research. Systematic errors like bias distort results in predictable ways, while random errors occur by chance and can be reduced through appropriate statistical methods. Confounding presents an additional challenge, as it can create false associations between exposure and outcome. Understanding these issues, along with the mathematical methods to detect and adjust for them, is crucial for producing valid and reliable research in epidemiology.
Humanities Moment
This articles featured image is The Mishap by Jan Van Chelminski (Polish, 1851 – 1925). Jan Chełmiński, also known as Jan van Chelminski, was a Polish painter born Jan Władysław Chełmiński. He began his artistic studies at the Munich Academy of Fine Arts on April 14, 1875, and worked across Europe. In 1895, he moved to New York, after obtaining British citizenship in 1893. Chełmiński gained widespread recognition during his lifetime for his historical paintings, particularly those focused on military history and the Napoleonic Wars.
Want to learn more about concepts in Epidemiology? Check our backlog of Epi Explained articles, as well as research summaries and R/Python tutorials!