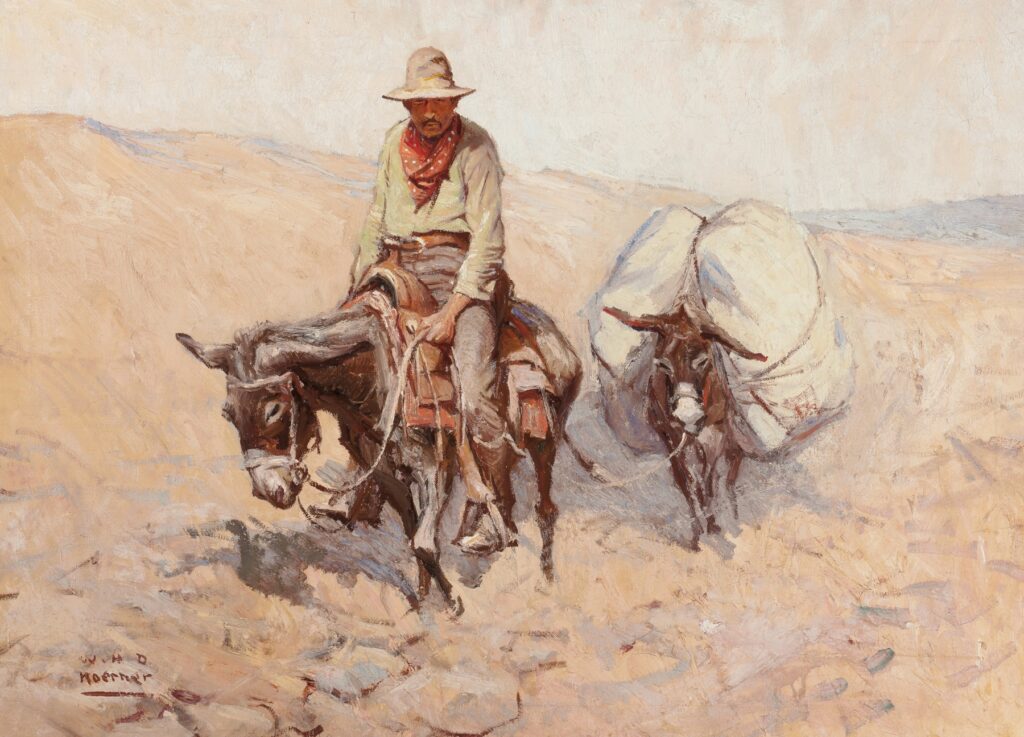
Epi Explained: What is Survival Analysis?
Survival analysis is a set of statistical methods used to analyze the expected duration of time until one or more events happen, such as the time until death, the occurrence of a disease, or the failure of a machine. This analysis is crucial in health research, clinical trials, and wider public health research because it helps estimate not only the likelihood of an event but also how long it may take for it to happen.
Key Questions
- What is survival analysis used for?
Survival analysis is used to estimate the time until an event occurs, such as death, disease relapse, or equipment failure. It is widely applied in healthcare, public health research, and social sciences. - What is censoring in survival analysis?
Censoring occurs when the event of interest hasn’t happened for some individuals during the study period. These individuals are included in the analysis but treated as incomplete data points. - What is the Kaplan-Meier estimator?
The Kaplan-Meier estimator is a non-parametric statistic used to estimate the survival function, giving insight into the probability of survival over time. - What is a hazard ratio?
A hazard ratio in the Cox model measures the relative risk of the event happening between two groups, with a ratio above 1 indicating higher risk and below 1 indicating lower risk.
Key Concepts of Survival Analysis
Several essential concepts form the backbone of survival analysis:
- Survival Time: The duration from the beginning of a study or a specific point in time until the event of interest (e.g., death or relapse).
- Censoring: This occurs when we do not observe the event of interest for some study participants during the observation period. Censored data points are still valuable but represent incomplete information.
- Hazard Rate: The hazard rate is the instantaneous risk of experiencing the event at a particular time, given survival up to that point.
- Survival Function: This function represents the probability that the event has not yet occurred by a specific time.
- Kaplan-Meier Estimate: A non-parametric statistic used to estimate the survival function from the time-to-event data.
Why is Survival Analysis Important?
Survival analysis is essential in fields like epidemiology and clinical research because it allows scientists to:
- Understand the distribution of survival times and how long patients or subjects live before experiencing an event.
- Estimate how different factors such as age, treatment type, and environmental exposure affect the probability and timing of the event.
- Make informed decisions about risk factors and treatment efficacy.
- Handle censored data effectively. In many studies, not all subjects experience the event, and survival analysis helps account for that.
Types of Survival Analysis Models
Several types of models are commonly used in survival analysis:
- Kaplan-Meier Estimator: Used for estimating the survival function without considering the impact of covariates.
- Cox Proportional Hazards Model: A semi-parametric model that assesses how the hazard rate depends on one or more covariates (such as age, gender, or treatment). It is particularly useful for adjusting for multiple risk factors.
- Parametric Models: These models assume the survival times follow a specific distribution (e.g., exponential or Weibull). They are useful when you have strong assumptions about the underlying survival process.
Common Applications of Survival Analysis
Survival analysis is used in a variety of fields:
- Medicine and Public Health: To study patient survival after diagnosis or treatment, or to examine the time to recovery, death, or relapse.
- Clinical Trials: To evaluate the effectiveness of new treatments or interventions in improving patient outcomes over time.
- Engineering/Occupational Public Health: In reliability engineering, survival analysis helps predict the time until equipment or system failure, which can help inform policies and guidelines around workplace equipment.
How to Interpret Survival Analysis Results
Understanding and interpreting survival analysis involves assessing two main outcomes:
- Survival Curves: These show the probability of surviving (event-free) over time. The Kaplan-Meier curve is the most common example, plotting the cumulative survival rate over the observation period.
- Hazard Ratios: In the Cox model, a hazard ratio quantifies the effect of a covariate on the hazard or risk of experiencing the event. A hazard ratio greater than 1 means higher risk, while less than 1 means lower risk.
Key Equations in Survival Analysis
Two of the most important equations in survival analysis is the survival function, often represented as:
[math]S(t) = P(T > t)[/math]
Where:
- S(t): Survival probability at time t.
- P(T > t): The probability that the event occurs after time t.
And the hazard function, which is often expressed as:
[math]h(t) = lim(Δt → 0) [P(t ≤ T < t + Δt | T ≥ t) / Δt][/math]
Where:
- : This is the random variable representing the time until the event occurs (e.g., time until death or failure).
- P(t≤T<t+Δt∣T≥t) : This term represents the conditional probability that the event occurs in the small time interval between t and t+Δt , given that the event has not occurred before time t (i.e., the subject has “survived” up to time Δt: This is a small increment of time.
- lim(Δt→0): This notation indicates that we are interested in the limit as Δt\Delta t approaches 0, meaning we’re looking at an instantaneous rate.
The hazard function, h(t), measures the instantaneous risk of the event occurring at time , given that the subject has survived up to that time. In other words, it’s the rate at which the event happens at a specific moment in time, conditioned on survival up to that moment.
Challenges in Survival Analysis
Survival analysis can be complex due to several challenges:
- Censoring: Dealing with data points where the event hasn’t occurred within the study period is difficult, but necessary for accuracy.
- Time-Dependent Covariates: If covariates change over time (e.g., a patient’s health improves or worsens), modeling them becomes more complex.
- Assumptions of Proportional Hazards: The Cox model assumes that the hazard ratio between two groups is constant over time, which may not hold in some cases.
Conclusion
Survival analysis is a vital tool in public health, medicine, and other fields where the timing of events is crucial to understand. It allows researchers to predict not only if an event will happen but also when it will happen, accounting for factors like patient characteristics, treatments, or environmental exposures. Whether you’re analyzing survival rates in clinical trials or system failure times in engineering, understanding survival analysis is essential for making data-driven decisions.
Humanities Moment
The featured image for this installment of Epi Explained is The Survival (1922) by William Henry Dethlef Koerner (American, 1878-1938). Wilhelm Heinrich Detlev “Big Bill” Körner was a renowned illustrator of the American West, gaining widespread recognition when his painting A Charge to Keep was featured on the cover of George W. Bush’s biography and displayed in the Oval Office. Born in Prussia and raised in Iowa, Koerner developed his artistic skills with minimal formal training before studying under influential artists like Howard Pyle. His career took off after illustrating Western-themed stories for major magazines like The Saturday Evening Post, producing nearly 2,000 works, including book illustrations for authors like Zane Grey. His bold use of color and dynamic depictions of the West placed him among the top illustrators of his time.
Thank you for reading this article of Epi Explained. If you’d like to learn more about modeling, epidemiology topics, or have any wider interest in Public Health, we’d love for you to check out the rest of our website.