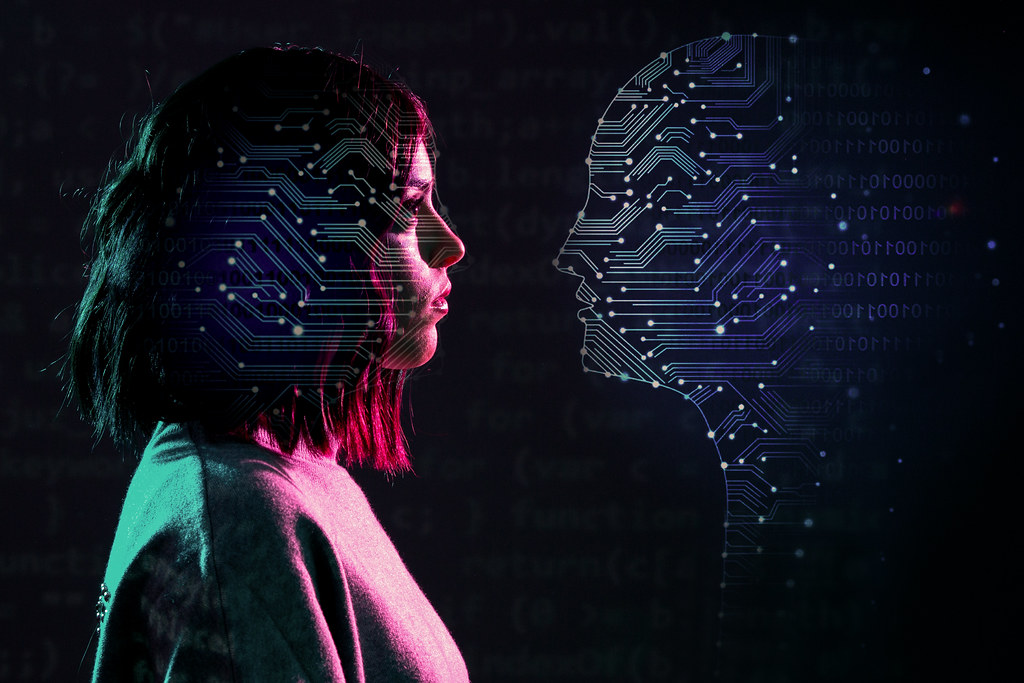
Inescapable AI: Public Health, Justice, and Algorithmic Accountability
Artificial Intelligence (AI) is reshaping critical systems like healthcare, housing, employment, and education, profoundly affecting low-income and marginalized populations. While AI holds immense promise, its unchecked application perpetuates systemic inequities and creates significant public health challenges. This article examines these dynamics, illustrating the far-reaching implications and offering strategies for reform based on a report created by TechTonic Justice called Inescapable AI.
AI as a Determinant of Public Health Outcomes
AI is no longer a distant abstraction; it is an integral force influencing the social determinants of health. In fact, it has been for decades but has reached a critical mass, and critical injection into, public life. Its deployment in areas such as Medicaid eligibility, tenant screening, and employment algorithms profoundly impacts who accesses essential resources. These systems, designed to optimize efficiency, often overlook the nuances of marginalized individuals’ lives, leading to unjust outcomes and a propagation of oppression under the guise of rationality.
For example, public benefits programs like Medicaid and the Supplemental Nutrition Assistance Program (SNAP) rely heavily on algorithmic systems. Over 73 million Americans depend on Medicaid, yet AI-driven systems have repeatedly flagged eligible individuals as ineligible due to minor discrepancies in their data. Similarly, 42 million SNAP beneficiaries face barriers when fraud detection algorithms falsely accuse them, cutting off vital food assistance. Such systems have also narrowed avenues for appeal for those who have been denied benefits, through automated chat systems and answer trees that are more optimized for the agency running the system, and not the community member using it.
What AI Means in the Public Health Context
In this context, Artificial Intelligence encompasses more than cutting-edge machine learning models. It includes any automated decision-making systems, from basic decision trees to complex neural networks, that analyze data to determine outcomes affecting individuals’ lives. AI’s decisions are often final, with limited human oversight, leading to disproportionate harm when these systems fail.
How Simple Decision Systems Have Shaped Analytics
Long before the rise of advanced AI like Gemini or ChatGPT, simpler decision-making systems like decision trees and regression models have been widely used in healthcare and public administration. These tools, when applied responsibly, provided actionable insights without the risks associated with “black box” algorithms. Decision trees, for instance, have been instrumental in clinical decision-making, guiding treatments based on transparent and interpretable rules. These methods are easy to employ, and we at Broadly Epi are working on tutorials for individuals to be able to build their own Machine Learning tools, with a focus on responsibility.
However, as technology advances, many systems are replaced by opaque algorithms that prioritize optimization over transparency. While decision trees might classify patients by risk levels based on clear factors, newer models often lack this interpretability, making it difficult to understand or challenge their decisions, especially if there is a disconnect between technical staff/contractors deploying these services, and decision makers who rely on them.
Key Areas of AI Impact
The report identifies several domains where AI is transforming decision-making, often with adverse consequences for marginalized groups.
1. Public Benefits
AI systems in public benefits administration exemplify how automation can unintentionally deepen inequities. With over 10.6 million people relying on Social Security Disability Insurance, the stakes are immense. AI often misjudges eligibility, denying benefits to those who need them most. Additionally, unemployment insurance systems using AI fraud detection have delayed aid for 1.1 million Americans, pushing many closer to financial precarity.
2. Housing
Housing insecurity, affecting 39.8 million renters, is exacerbated by AI-driven tenant screening. These systems frequently use outdated or incorrect data, unfairly rejecting applications. Algorithms that set rents often prioritize profit over affordability, further destabilizing low-income communities. Likewise, data biases, particularly around age, gender, race and education, can all cause applicants for housing that are more than capable to be denied outright, through only the fault of the chance of who they were born as, and not who they are.
3. Employment
In the labor market, AI determines who gets hired, how much they are paid, and their working conditions. Gig economy platforms heavily rely on algorithmic wage-setting and worker management, which often exploit laborers. Additionally, as has been seen in many reports and articles, employment screening AI systems tend to reinforce existing biases around positions. For instance, Amazon, a notable titan in the AI and Tech world, ended up having to shut down an AI screening tool as after evaluation it was shown to have a strong bias against women. For the 32.4 million Americans in low-wage jobs, this creates a cycle of instability and limited upward mobility, especially for those trying to push historical occupational boundaries.
4. Education
In schools, predictive AI systems identify “at-risk” students, disproportionately labeling marginalized children. Approximately 13.25 million students face the effects of such misclassification, which can stigmatize them and reduce their access to educational opportunities.
5. Domestic Violence and Child Welfare
AI’s impact on domestic violence and child welfare systems is particularly concerning. Predictive tools often fail to protect survivors adequately or misclassify risk, contributing to family separations and inadequate interventions.
The Double-Edged Sword of AI in Public Health
AI has the potential to revolutionize public health by automating labor-intensive tasks, identifying disease patterns, and tailoring interventions. For instance, AI can analyze death records to assess trends in violence or evaluate large datasets to inform public health campaigns. However, these benefits hinge on ethical application and robust oversight.
Without transparency, AI systems risk entrenching systemic inequities. Algorithms trained on biased data often replicate and amplify those biases, disproportionately harming marginalized groups. This is particularly dangerous in contexts like housing and employment, where discriminatory practices have long histories.
Recommendations for Equitable AI Use
To mitigate harm and harness AI’s potential, the following actions are essential:
- Community Empowerment: Invest in community education to help individuals understand and challenge AI-driven decisions.
- Regulatory Oversight: Implement policies ensuring transparency, accountability, and fairness in AI use. For instance, tenant screening algorithms should undergo regular audits to ensure accuracy and fairness.
- Responsible Design: Develop AI systems that prioritize equity, such as tools to automate benefit enrollment rather than penalize recipients. Furthermore, advancing user or customer centered design, as opposed to pure efficiency, would likely be a boon as opposed to a barrier. One such example may be something as simple as for SNAP or Medicaid benefits, allowing users to snap a picture of their information, and using OCR (Object Character Recognition) to extract key data, confirm it with the user, and then submit what’s required, with a rejection automatically going to a human for appeal with rationale for why the AI’s decision should be overturned. This would take excess burden off the applicant, and ensure proper oversight of systems.
- Ethical Guidelines: Establish ethical standards for AI in public health, focusing on reducing disparities and improving access to resources.
Conclusion: A Call to Action
AI is reshaping how society delivers critical services, but its unchecked application risks deepening systemic inequities. By prioritizing transparency, accountability, and community engagement, we can redirect AI’s trajectory to support equitable public health outcomes. Without redirection, we risk falling futher into dystopian, misanthropic societies in which those we claim to help as public health professionals are only harmed more by our, and others, inaction. The stakes are high, but so is the opportunity to build systems that work for everyone. We can build that opportunity with better education, better oversight, and more respect for the agency of the people we serve and work alongside every single day.
The featured image for this article was sourced from Flikr, created by Jérémy Barande, and used under a Creative Commons 2.0 License.