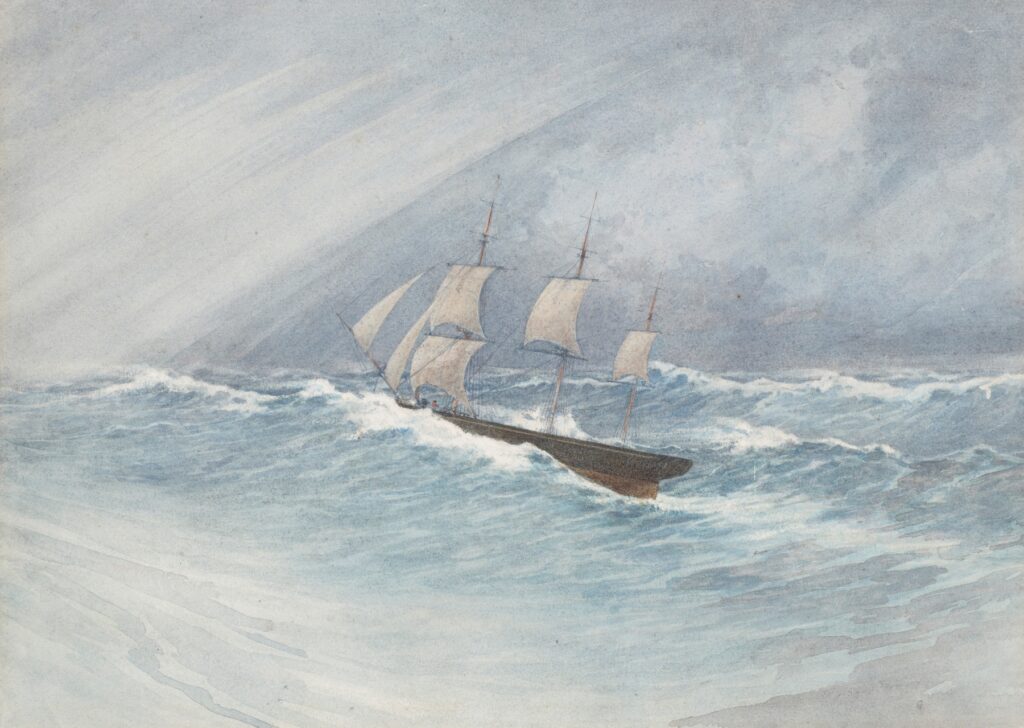
Evidence-Based Approaches to Public Health: Epidemiology – Bias and Confounding: Confounding Variables and Effect Modification
In this tutorial, we will explore two critical concepts in epidemiology: confounding variables and effect modification. Confounding occurs when an outside factor distorts the relationship between an exposure and an outcome, while effect modification occurs when the effect of an exposure on an outcome differs depending on a third variable. Understanding these concepts is essential for conducting accurate public health research and for the Certified in Public Health (CPH) exam.
By the end of this tutorial, you will understand what confounding and effect modification are, how they affect epidemiological studies, and how to control for confounding and identify effect modification. Practice questions are provided to reinforce your knowledge.
Table of Contents:
- Introduction to Confounding and Effect Modification
- Confounding Variables
- Definition of Confounding Variables
- Examples of Confounding
- Methods to Control for Confounding
- Effect Modification
- Definition of Effect Modification
- Examples of Effect Modification
- How to Identify Effect Modification
- Practice Questions
- Conclusion
1. Introduction to Confounding and Effect Modification
Both confounding and effect modification are important concepts in epidemiology that describe different ways in which third variables can influence the relationship between an exposure and an outcome. Confounding can distort the true relationship between exposure and outcome, leading to incorrect conclusions, while effect modification (also known as interaction) occurs when the effect of an exposure varies depending on the level of another variable.
Distinguishing between confounding and effect modification is critical for interpreting research results accurately and making valid public health conclusions.
2. Confounding Variables
A confounding variable (or confounder) is a third variable that is related to both the exposure and the outcome, which can distort the observed relationship between them. Confounding can make an association between an exposure and an outcome appear stronger, weaker, or even reverse the direction of the association.
2.1 Definition of Confounding Variables
Confounding occurs when a third variable influences both the exposure and the outcome, leading to a spurious association. For a variable to be a confounder, it must meet the following criteria:
- The confounding variable must be associated with the exposure.
- The confounding variable must be associated with the outcome, independently of the exposure.
- The confounding variable should not be on the causal pathway between the exposure and the outcome.
2.2 Examples of Confounding
Let’s explore a few examples of confounding:
- Example 1: A study examines the relationship between alcohol consumption and heart disease. Age could be a confounder if older individuals are more likely to consume alcohol and are also at higher risk for heart disease.
- Example 2: A study investigates the association between physical activity and diabetes. Socioeconomic status (SES) may be a confounder if individuals with higher SES are more likely to exercise and have lower rates of diabetes.
2.3 Methods to Control for Confounding
Several methods can be used to control for confounding in epidemiological studies:
- Randomization: In experimental studies, random assignment of participants to exposure groups can help ensure that potential confounders are evenly distributed between groups.
- Matching: In case-control studies, matching cases and controls on potential confounders (such as age or sex) ensures that these factors are equally distributed across groups.
- Stratification: Analyzing data within strata of the confounding variable can help isolate the relationship between the exposure and the outcome without the influence of the confounder.
- Multivariable regression: In observational studies, using multivariable regression models allows researchers to adjust for confounders by including them as covariates in the analysis.
3. Effect Modification
Effect modification occurs when the effect of an exposure on an outcome varies depending on the level of another variable. Unlike confounding, effect modification is not a bias but rather an important finding that reveals how different groups are affected differently by the same exposure.
3.1 Definition of Effect Modification
Effect modification (also called interaction) occurs when the relationship between an exposure and an outcome changes depending on the presence or level of a third variable. The effect modifier is a variable that alters the strength or direction of the association between the exposure and the outcome.
3.2 Examples of Effect Modification
- Example 1: A study looks at the association between smoking and lung cancer. The risk of lung cancer might be higher in older individuals compared to younger individuals who smoke, meaning age acts as an effect modifier.
- Example 2: A study investigates the relationship between exercise and heart disease. The protective effect of exercise may be stronger among individuals with a family history of heart disease, indicating that family history is an effect modifier.
3.3 How to Identify Effect Modification
Effect modification is identified by analyzing the data in subgroups of the potential effect modifier and comparing the strength or direction of the association between the exposure and outcome across these subgroups. If the effect of the exposure differs across subgroups, then effect modification is present.
Steps to identify effect modification:
- Stratify the data by levels of the potential effect modifier (e.g., age groups, sex).
- Compare the association between exposure and outcome in each stratum.
- If the strength or direction of the association differs across strata, effect modification is present.
Unlike confounding, effect modification is not something to control for, but rather something to report and understand as it reveals important differences in how various groups respond to the exposure.
4. Practice Questions
Test your understanding of confounding and effect modification with these practice questions. Try answering them before checking the solutions.
Question 1:
A study investigates the relationship between physical inactivity and obesity. Age is associated with both physical inactivity and obesity but is not part of the causal pathway. What is age in this scenario?
Answer 1:
Answer, click to reveal
Age is a confounder because it is associated with both the exposure (physical inactivity) and the outcome (obesity) but is not part of the causal pathway between them.
Question 2:
A study examines the effect of air pollution on respiratory disease. The effect of air pollution on respiratory disease is found to be stronger in individuals with asthma than those without asthma. What is asthma in this scenario?
Answer 2:
Answer, click to reveal
Asthma is an effect modifier because the relationship between air pollution and respiratory disease varies depending on whether an individual has asthma.
Question 3:
In a study on the relationship between high blood pressure and stroke, researchers control for age and sex by including them as covariates in a regression model. What method are the researchers using to control for confounding?
Answer 3:
Answer, click to reveal
The researchers are using multivariable regression to control for confounding by adjusting for age and sex in the analysis.
5. Conclusion
Understanding confounding variables and effect modification is essential for conducting and interpreting epidemiological research accurately. While confounding can distort the observed relationship between an exposure and an outcome, effect modification highlights how the effect of an exposure varies across different subgroups. Recognizing and accounting for these factors ensures that research findings are valid and informative for public health interventions.
Remember:
- Confounding occurs when a third variable influences both the exposure and the outcome, leading to a distorted association. It can be controlled using methods such as randomization, matching, stratification, or multivariable regression.
- Effect modification occurs when the effect of an exposure on an outcome varies across levels of another variable. It is not a bias and should be reported to understand how different groups are affected by the exposure.
Final Tip for the CPH Exam:
Ensure you understand the differences between confounding and effect modification, and look more into methods like multivariable regression analysis. Knowing how to control for confounding and report effect modification will be crucial for success on the Certified in Public Health (CPH) exam.
Humanities Moment
The featured image for this article is Old style ship, ‘Kenilworth’ (1857) by James Crowe Richmond (New Zealander, 1822 – 1898). Richmond was a New Zealand politician, engineer, and early watercolorist who captured the New Zealand landscape. Born in London, he worked under Isambard Kingdom Brunel before emigrating to New Zealand in 1850, where he settled in Taranaki and later Nelson. Richmond served in Parliament, held various provincial roles, and maintained a lifelong passion for painting, forming a friendship with artist John Gully. Despite personal tragedies, including the loss of his wife, he continued to travel, contribute to politics, and influence New Zealand’s artistic heritage through his work and his artist daughter, Dorothy Kate Richmond.